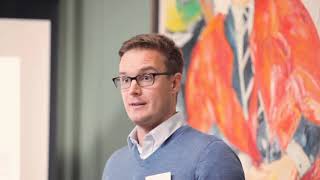
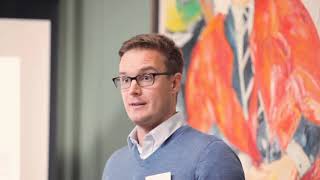
Data-Centric Engineering: A new language for 21st century civil engineers
At the 2017 Annual CSIC Industry partners Garden Party at Peterhouse College, Cambridge, I discussed the new emerging field of...
Assistant Professor (Structures/Materials Engineering)
Department of Civil Engineering
Lassonde School of Engineering
York University, Toronto, Canada
Regular visual and tactile inspections form the basis for informing management decisions, establishing remaining capacity and ensuring the safety bridges around the world. In the UK, bridge inspections represent a multi-million pound investment by both highways and railway authorities. Aside from their cost, bridge inspections can place operators at risk, only provide condition-based information (i.e. no quantitative link to remaining capacity), and may not capture the condition of critical elements which are either hidden or inaccessible.
The challenge is to develop monitoring systems and data analysis tools capable of providing the information required to assess structural capability remotely and in real-time, thereby significantly reducing the frequency of tactile inspections of bridges. A secondary benefit is being able to assess in real time the effect of increased or abnormal loads on the structure which could inform points of intervention and reduce the need for additional repair or strengthening. Integrated monitoring therefore enables both a proactive and reactive approach to bridge asset management.
An Innovate UK and EPSRC-funded research project in collaboration with the Staffordshire Alliance (Network Rail, Laing O'Rourke, Atkins, and VolkerRail) and the University of Cambridge's Centre for Smart Infrastructure and Construction (CSIC) invested to create the UK's first prototype 'self-sensing' railway bridges. Two of the 11 new bridges forming part of the SAIP (one steel half-through and one prestressed concrete girder) were extensively instrumented with a durable, permanent, fibre-optic based strain monitoring system. Monitoring data was captured during the construction of the bridges up until they were placed in service in April 2016.
This project will develop the capability and tools required to deliver ongoing and remote assessment of the structural condition and remaining capacity of bridges and will provide a portable framework for planning of future smart infrastructure management projects.
The field of structural health monitoring is reaching maturity as developments in sensor technology, data analytics, wireless communication and automated asset management techniques are advancing rapidly and being applied to monitor and assess new and aging infrastructure. Missing from current capabilities is the ability to effectively gather smarter information on the performance of deficient structures which have been retrofitted. By combining sensing and strengthening techniques, a hybrid ‘dual-purpose sensing’ system could be used to design more effective retrofits, provide information on long-term performance, and improve overall resilience.
An experimental programme is currently underway at the University of Cambridge to develop a proof-of-concept for smart 'Band-Aids' which integrate fibre optic sensing (FOS) within a fabric-reinforced cementitious matrix (FRCM) for use in the strengthening and monitoring of critical concrete infrastructure such as bridges, buildings, containment vessels, etc. It is envisioned that future research will involved full-scale field implementation of the proposed system on in-service concrete structures.
This research area evolved from completing my PhD at the University of Waterloo in 2012 under the direction of Prof. Jeffrey S. West and Prof. Susan L. Tighe. My PhD research involved extensive testing of reinforced concrete specimens for the purpose of implementing new testing methods and developing classification systems for the use of structural recycled concrete. Specifically, reinforcement bond strength was measured using beam-end specimens in order to determine the effect that replacing natural aggregate with various recycled concrete aggregates had on the bond strength and bond-slip mechanisms in reinforced concrete members. To measure displacement, beam-end specimens were instrumented with sets of linear variable differential transformers and a servo-hydraulic testing system was used to facilitate a displacement-based rate of loading. Through this work I succeeded in developing regression-based equations for assessing how a particular recycled concrete aggregate type influences the development length of reinforcing steel.
Another component of the research involved measuring the fracture energy of recycled concrete. I developed a new test specimen arrangement called a single-edge notched double cantilevered prism to derive the load-displacement curve used to calculate the fracture energy. Due to the highly-sensitive nature of capturing the entire load-displacement behaviour of plain concrete, a closed-loop servo-hydraulic testing system was employed and the crack mouth opening displacement, measured using a clip gauge, was used as the control channel. This test method proved to be highly successful as it was able to capture the entire post-peak behaviour of the concrete specimens and allowed for accurate calculation of the fracture energy. Based on the results, regression models were developed to provide statistical relationships between the fracture energy and bond strength of recycled concrete. An electronic copy of my PhD dissertation may be accessed here.
My current research interests in sustainble concrete materials have maintained my involvement as a voting member on ACI committee 555 on Recycled Concrete Materials where I have been working to update the committee's flagship report on the Recycling and Reuse of Concrete. I am also currently exploring other research opportunities relating to other forms of low-carbon concrete as well as self-sensing and self-healing cementitious based materials.
The emerging field of Data-Centric Engineering (DCE) is a synthesis of approaches for studying physical engineering assets which leverages both physics-based and statistical models. Through a partnership between the Alan Turing Institute and the Lloyd's Register Foundation, a research programme in Data-Centric Engineering (DCE) was established in 2015.
Through the Cambridge Centre for Smart Infrastructure and Construction (CSIC), I became involved with the DCE programme in April 2017 through a project on Instrumented Infrastructure. This project has involved analysing monitoring data collected from sensor networks that were previously installed by the CSIC on several new and existing railway bridges in the U.K (refer to work on self-sensing bridges). The primary goal of this research is to leverage advanced statistical and machine learning techniques to improve the interpretation of structural health monitoring data. A secondary goal is to develop 'digital twin' bridge models (i.e. cyber-physical systems) which will exist alongside the physical assets and be updated via a statistical process as new data is collected. These DCE-enabled models can then be used to perform 'what-if' scenarios providing asset managers with a powerful tool for assessing long-term risk.
Since March 2018, I have been cross-appointed as a Group Leader within the DCE programme working with Prof. Mark Girolami and a number of other research collaborators at the Alan Turing Institute and the University of Cambridge. My future research in this area opens up many avenues of application. I am particularly interested in how DCE approaches can be applied not only to help better understand instrumented infrastructure but also for accelerating development of next-generation construction materials and improving the overall resilience of our built environment.
The rail network represents a critical piece of infrastructure in the U.K. and in many other countries around the world. It requires constant maintenance, planning and innovation to ensure that its future performance is adequately safeguarded. A critical component of the rail network is the track bed system itself which comprises the subgrade, ballast, sleepers, fasteners and rails. In particular, the sleepers are the primary structural components which carry the wheel loads from the rolling stock, to the rails and into the ballast. Sleepers are primarily designed for dynamic loads, and the way in which the dynamic loads are distributed into the supporting ballast is highly dependent on the condition of the ballast itself. Furthermore, the sleeper materials and their manufacturing process can have considerable influence on both their short- and longterm performance. Therefore, monitoring sleepers throughout their entire life would provide invaluable information to rail asset managers on the performance of this critical component of the rail network.
This study is related to my research work on self-sensing railway bridges as several prestressed concrete sleepers instrumented with fibre optic sensors have been installed on these bridges. In partnership with Heriot-Watt University, a series of full-scale experimental tests of instrumented sleepers on ballast are being conducted. The results of these tests are currently being studied in order to evaluate the robustness of the self-sensing sleepers, to correlate strain response to differential ballast settlement, axle load and ballast pressure, and to develop/validate nonlinear finite element models as tools for interpreting long-term field monitoring data collected from self-sensing sleepers.